top of page
ADELE MYERS
Adele Myers CV
I develop interpretable AI tools to quantify complex shape changes in the human body. I am specifically interested in using these tools to study shape changes that happen in the female brain during hormonal events such as menstruation, pregnancy, and menopause. Shape analysis tools are immensely powerful in this area. While complex 3D shape changes in the brain are easily noticed by the human eye, the specific characteristics of the shape change cannot be quantified by the human eye. My work seeks to quantify subtle changes, giving doctors interpretable information about the nature of the shape change and informing diagnoses and research.
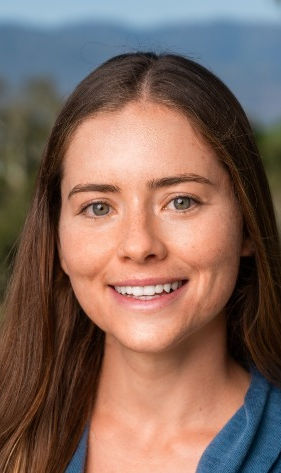
bottom of page